A Roadmap for Tax Assessor Office “Explainability”
Einstein once said, “If you can’t explain it simply – you don’t understand it well enough.” Perhaps nowhere on Earth does this nugget of wisdom ring truer than an assessor’s office being successfully challenged over updated property valuations. Of course, a tax assessor’s best insurance against that dilemma is clear, data-backed evidence that valuations are generated through stringent due diligence, and are rooted in meaningful reality. In a word: “Explainability.”
We’ve had a lot to say about Responsible AI for the tax assessment realm in recent months: Prioritizing privacy and safety in AI development—we’ve committed to help tax assessors navigate this new technology with a focus on accuracy, equity, efficiency, productivity, transparency and accountability. Taking a holistic look at our commitment, the bedrock of all these goals is explainable, high-fidelity data. The aim of this blog post is to put technology in context around the core foundation of Responsible AI for tax assessors: A highly focused, scientific practice that I call Data Stewardship.
What is Data Stewardship?
While standards certainly exist—the reality is that data curation, measurement and analysis practices can vary widely between states, counties and individual assessor offices. In the end, data quality suffers. Factor in the varying degrees of government investment in assessor staffing levels and assessment processes, and disparate ratios of primary field research to third party data—and valuation consistency within a single county becomes aspirational at best. Add to it the inescapable reality that human error exists, and it’s clear that adopting a more scientific approach is necessary.
Just as a pharmaceutical company wouldn’t dream of releasing a drug without conducting a comprehensive series of clinical trials—encouraging patients everywhere to “just trust us on this one,”—tax assessors need to be responsible stewards of the data they use to generate property values, and continuously strive to improve data quality. Just like any other science, the rigor behind the entire life cycle of tax assessment data—from curation to analysis and output to ongoing review—must increase, treating data as a product unto itself.
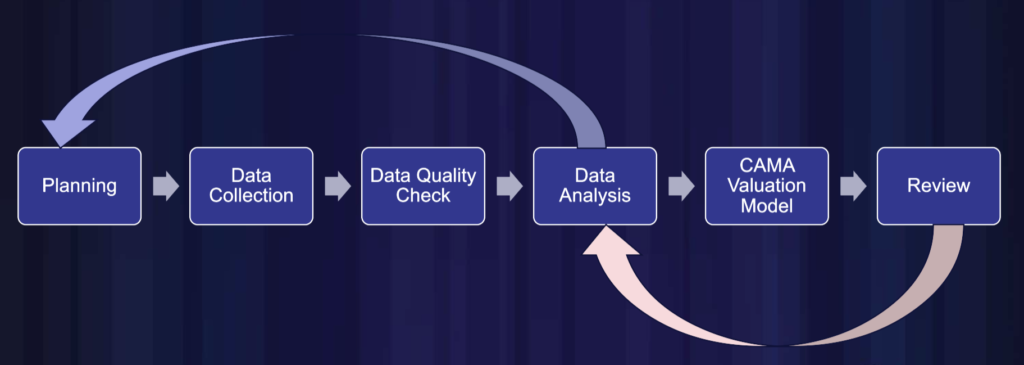
The Three Pillars of Data Science
So, how can tax assessors continuously improve data quality that fuels defensible valuations? To start, by simply going against the grain. As a rule, humans manipulate data to draw the inferences they want to draw. True data science doesn’t do that. It recognizes the role and utility of three discrete processes that lead to higher fidelity data.
Distinct from the narrower confines of data engineering and generative AI, data science is fueled first by a commitment to Data Stewardship, then rigorous statistical analysis, followed by machine learning.
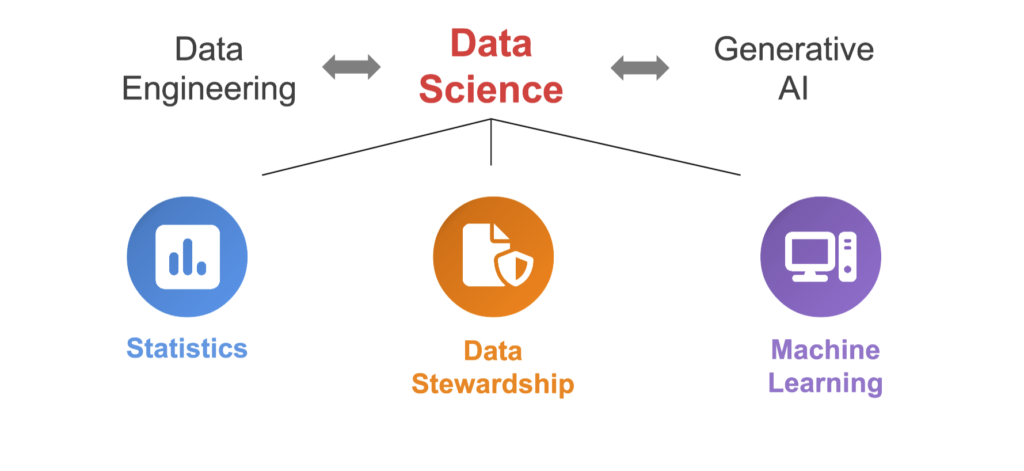
Data Stewardship
The linchpin of data science, Data Stewardship is concerned with the entire life cycle of data and the integrity around it. From the strategic planning of discrete data to be collected, and the approach and timing of its curation, to its examination for adverse influence and ongoing analysis, to its final reported output—Data Stewardship considers, selects, shepherds, analyzes and continually tests and improves data with the same rigor a scientist in any other field would.
Statistics
In use for decades, and the core component of data engineering—statistics remains the gold standard for property valuation. However, regression analysis is something most assessors generally don’t leverage. 15-20% of assessors who do, may manually perform this analysis in Excel—and only once or twice in an entire assessment cycle.
Machine Learning
A core component of generative AI, Machine Learning (ML) is making use of statistics easier, faster and more affordable. With the capacity to curate real time sales data, and the computing power to completely automate regression analysis—ML models equip assessors to improve data quality and perform statistical analysis on a daily basis.
The Limits of AI to Advance “Explainability”
While machine learning can radically enhance assessors’ ability to augment and improve data quality in real time using sales data—it’s important to recognize that not every aspect of AI will be useful for increasing assessor office efficacy. Take for example large language models (LLM). While this ML model has garnered the most attention for its ability to comprehend and generate human language text, it adds very little value to assessors as it’s been trained on volumes of knowledge completely irrelevant to them and their content objectives.
In a similar vein, leveraged indiscriminately—some elements of AI can be potentially dangerous. Beware of feeding your own data to LLM, lest it become public property. If an LLM is hacked, would-be attackers could conceivably access personal data it has been trained on, and use it to commit identity theft, fraud, or other crimes.
For all its power and capacity to support Data Stewardship for tax assessors—it’s also important to note that until recently, ML model algorithms haven’t been entirely transparent. This signals the need to develop ML methods that are more explainable under the principles of Responsible AI—an industry challenge we’re excited to rise to the occasion of, and collaboratively solve with our customers.
What explainability gaps do you see in the assessment cycle that beg for better Data Stewardship and technology solutions? Let us know!